Artificial intelligence (AI) has been making headlines everywhere—from individuals using ChatGPT for day-to-day tasks, to companies developing their own AI engines. However, it is often overlooked that AI and machine learning (ML) have been around for quite some time, with many real-world applications preceding ChatGPT’s release.
When it is not through a chat, sometimes we might be unable to identify if what we see is calculated through AI-driven processes, as these systems fundamentally depend on statistical and mathematical models.
In this article you’re going to see some real examples of what AI means for energy management, including our new forecasting module, and you have the opportunity to sign up to our upcoming webinar.
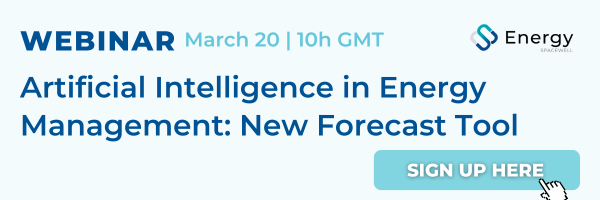
Machine learning and AI in energy management
One of the simplest forms of ML is a linear regression model—y = Ax + B—familiar to most engineers. As you might imagine, the discipline extends to a wide variety of more sophisticated approaches, including neural networks.
Taking this into account, how is artificial intelligence linked to energy management?
The energy management sector can greatly benefit from AI-driven solutions. Among the key areas where AI models prove essential are:
- Baseline creation to comply with protocols such as the International Performance Measurement and Verification Protocol (IPMVP) to demonstrate energy savings.
- Forecasting energy consumption or production.
- Detecting anomalies and inefficiencies in buildings.
- Learning building behaviour under specific ambient conditions.
Given these diverse use cases have existed for many years and have often been tackled through simple models, a pertinent question arises: when should we opt for those simple models like linear regressions and when should we adopt more advanced approaches?
Certain regulations often dictate the use of specific models. Under IPMVP guidelines, for example, multiple linear models (Ax+By+Cz+…+D) must be employed to quantify savings, even if other methods might yield superior results, i.e. no advanced machine learning is allowed yet. To facilitate this process, Spacewell Energy developed the ABC App, which helps streamline the creation of such equations.
However, when there is no legislative restriction, it is essential to balance accuracy and the resources invested. For instance, it would be inefficient to use a highly complex model to explain a correlation between two variables, such as checking if there is a correlation between heating degree days (DD) and electricity consumption. Instead, more advanced models become worthwhile when the relation between inputs and outputs is not straightforward, for example in energy forecasting.
Notwithstanding, some customers have expressed concerns about not being able to control the model that is calculating results. With a simple baseline, they can choose the inputs, they can calibrate the models themselves and they can easily see the effect a change in the inputs will cause to the outputs. Instead, with complex ML models this is not possible, since it sometimes works as a black box. As the models themselves might have multiple dimensions, they cannot be represented graphically or through an equation and sometimes it is hard to know the effect of each input to the output.
Therefore, in Spacewell Energy we apply advanced machine learning in those use cases where it is required to solve a specific problem or it significantly delivers better results.
A practical example: New Forecasting tool in Spacewell Energy
Spacewell Energy recently launched a forecasting tool capable of predicting resource consumption and production (electricity, gas, water) for the next 10 days, incorporating meteorological data.
Taking into account all the information that has been shared in the previous section, we wanted to see the difference in results between simple and complex models. To do so, we compared four prediction methods across 12 months:
- Simplest: Linear Regression (Ax + By + Cz + (…) + D)
- Polynomial Regression (Ax + Bxy + Cz2 + (…) + D)
- Most complex: Two more complex AI models that are offered within our forecasting tool:
- Best-performing AI model in the tool (the one showing the lowest error).
- Worst-performing AI model in the tool (the one showing the highest error).
Results showed that the best-performing AI model provided a 28% reduction in prediction errors compared to other methods. Moreover, it was the model providing the best prediction in all of the 12 months where the four models were evaluated:
The percentage of error might not help you to understand how much better one model is vs. the other. To be clearer, we also showed the graphical difference between the models. Below you can observe the difference between the best-performing AI model and the others, being the first one the only able to follow the real consumption curve:
In this case, we decided to use the complex AI models since the results they were providing were significantly better than the simpler models.
Other applications of Artificial Intelligence in Energy Management
In Spacewell Energy we not only have the forecasting feature but we also have more AI-based products:
- Optimise: our anomaly detection engine
- Detect: our benchmarking tool that helps identify low-hanging fruits within a company’s portfolio
- Power optimisation (in Spain only at the moment): Our tool that helps users identify the best contracted power for their buildings to minimise power costs
- ABC (Automatic Baseline Creation): Our tool that helps create baselines that are compatible with the IPMVP protocol.
You can learn more about our forecasting tool, Optimise, Detect and ABC during our next webinar on March 20.
AI has been part of the energy management landscape for far longer than many people realise, underpinning everything from baseline creation to anomaly detection. Although regulations such as IPMVP necessitate simpler methods like multiple linear regression, there is abundant evidence that more complex models can outperform these traditional approaches when there is no legislative constraint. Balancing the complexity of these solutions with the need for transparency remains a key challenge, as advanced machine learning models are often difficult to visualise and interpret.
Nonetheless, real-world outcomes illustrate the clear benefits of embracing AI-driven innovation. Spacewell Energy’s forecasting tool, for instance, demonstrated a notable 28% improvement in prediction accuracy when using a more advanced model instead of simpler methods. While some users may initially hesitate to adopt solutions they cannot fully deconstruct, the marked gains in efficiency and reliability confirm that, in many cases, these state-of-the-art AI techniques offer the most effective path forward.
To continue talking about AI in energy management and the new Forecast feature, we are hosting a new webinar on March 20. Sign up today to stay up with the latest trends and innovation.
Note of the Editor: This article has been written by Júlia Bayascas, Product Manager at Spacewell Energy.